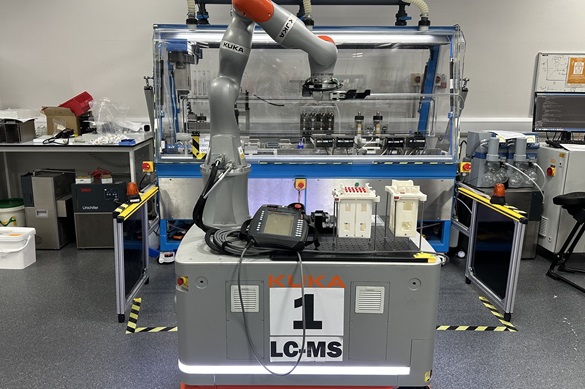
AI-driven robots for decision-making
Submitted by:
Andrew Warmington
Professor Andrew Cooper’s team at the University of Liverpool’s Department of Chemistry and Materials Innovation Factory have published the results of a study in Nature*, showing that mobile robots that use AI logic to make decisions were able to perform exploratory chemistry research tasks much faster than humans and to the same level.
The team designed and used two 1.75-meter-tall mobile robots to tackle three primary problems in exploratory chemistry: performing the reactions, analysing the products and deciding what to do next based on the data. The work thus addressed three different areas of chemical synthesis of structural diversification chemistry, supramolecular host-guest chemistry, and photochemical synthesis.
Post-doc researcher Dr Sriram Vijayakrishnan, who led the synthesis work, noted that collecting and working out analytical data can take as long as setting up experiments. “This problem becomes even more severe when you start to automate the chemistry. You can end up drowning in data.”
Cooper added that decision-making can be at least as time-consuming as the parts of the process that are more typically automated, especially in exploratory chemistry, where the outcome is uncertain. “It involves subtle, contextual decisions about whether something is interesting or not, based on multiple datasets.”
By using AI logic, the robots can process analytical datasets to make an autonomous decision instantaneously, whereas a chemist would take hour to go through the same datasets. They can evaluate multiple contexts, such as novelty of the reaction product, or the cost and complexity of the synthetic route.
Although they lack the contextual breadth of a trained researcher, Cooper said, “the AI logic made more or less the same decisions as a synthetic chemist across these three different chemistry problems in the blink of an eye. There is also huge scope to expand the contextual understanding of the AI, for example by using large language models to link it directly to relevant scientific literature.”
The team now aims to use this technology to discover chemical reactions relevant to drug synthesis, as well as new materials for applications like carbon capture. The process can be scaled to the largest industrial uses as there is no limit to the size of robot teams that could be used.
* - Nature, 635, 890–897 (2024): doi: 10.1038/s41586-024-08173-7