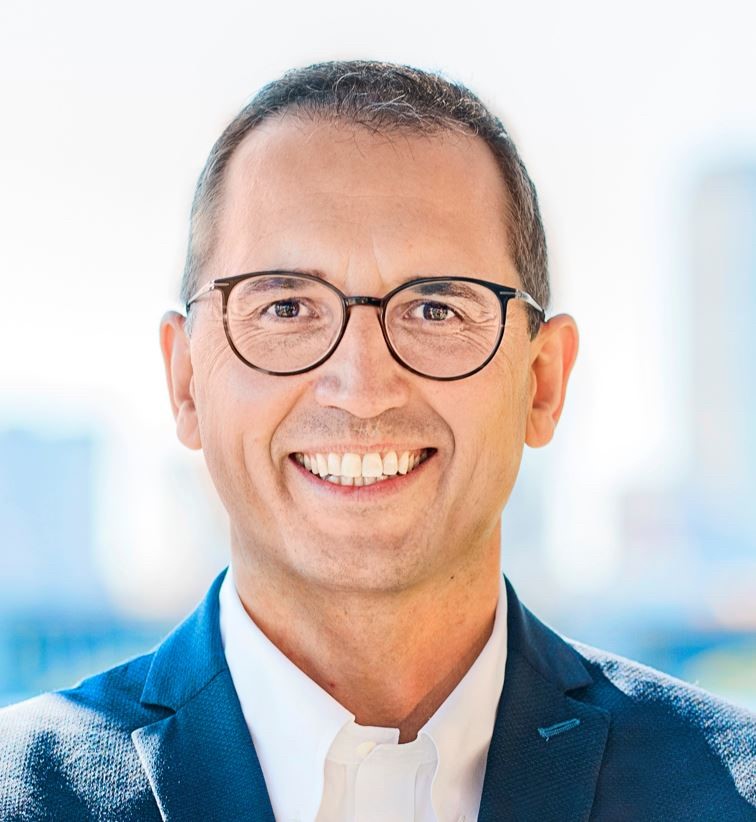
Capture tacit workforce knowledge - before it’s too late
Submitted by:
Andrew Warmington
A centralised knowledge management system can help chemical manufacturers retain valuable tacit knowledge from retiring or exiting workers, according to Andreas Eschbach, CEO and founder of Eschbach
Over the next five years, up to 25% of the process manufacturing workforce could be eligible for retirement. At the same time, younger workers are shifting jobs more frequently. These changes in the workforce leave manufacturers at risk of losing valuable historical knowledge and experience. It also makes it difficult for the next generation of workers to build the deep institutional knowledge necessary for smooth operations.
This is the tacit knowledge problem. Tacit knowledge is the ‘on-the-job’ knowledge gained through experience and observation; it is often undocumented, hidden and unrecognised until it is needed. It also includes institution-specific history and ways of doing things.
For example, a long-time maintenance director may be able to diagnose and troubleshoot a problem quickly with a piece of equipment based on history and experience that goes beyond what is documented in the owner’s manual. Or an experienced process engineer may know that a formulation has to be tweaked at certain times of year to account for environmental conditions.
Tacit knowledge can be difficult to articulate and document, but its loss can have direct impacts on plant safety, product quality and operational efficiency. As experienced workers leave the workforce through retirement or job changes, knowledge that exists outside of formal documentation and SOPs can be lost. Manufacturers need a systematic way to collect, preserve and communicate tacit knowledge so future employees can benefit from the experience of their predecessors.
Knowledge transfer through digitalisation
Knowledge transfer is the sharing of knowledge between individuals and groups in an organisation. It can be formal (e.g. training and SOPs) or informal (an experienced colleague showing you the ropes). The knowledge transfer process consists of: *
Preserving existing knowledge and data
* Generating the right information when it is needed
* Communicating that information to the right people at the right time in a form they can use
* Applying knowledge to the situation at hand Most tacit knowledge is shared informally
The challenge, especially with a rapidly shifting workforce, is capturing this tacit knowledge in a systematic way and making it findable and usable when it is needed. This is where a centralised, digital knowledge management system comes in. It starts with digitalisation and centralisation.
Maintenance logs, shift handover notes, inspection observations, and ad hoc communication between employees (e.g. via email or instant messaging apps) contain a wealth of data and historical knowledge that exists outside of formal SOPs and training programs. However, in most plants, these forms of knowledge are scattered across many platforms and systems, including manual notes and offline conversations.
To capture this knowledge, we need to bring everything into one centralised system, where it can be easily stored, searched, shared and analysed. This requires both technological and cultural shifts in the organisation. Data that were once offline or in isolated systems—such as shift handover notes, manual inspection observations, maintenance logs or daily work assignments—need to be put into a digital format in a common platform.
A digital plant process management (PPM) system acts as a centralised knowledge capture system, collecting everything in one place. Employees must get used to logging their activities, observations, notes, and communications in the PPM. Human-generated data is combined with automated data from sensors and equipment to provide a holistic and comprehensive view of the plant.
Capture is only step one, however. In addition to preserving data and knowledge, we also need to make it findable and usable in the future.
In a chemical manufacturing plant that generates thousands of data points every hour, it can be a challenge to find patterns hiding in the data or historical information that is relevant to a current problem. A knowledge management system includes search and analytical tools that make it possible to deliver the right information, at the right time, to the right people.
Building a people-centred knowledge platform
State-of-the-art knowledge management platforms are not just about data. They are about people and how those people will use the data. Emerging artificial intelligence (AI) tools can make these systems more people centric.
For example, natural language processing (NPL) allows people to interact with the system using plain language: instead of searching through the system, they can simply ask, “Has this product colour ever changed to grey?” Machine learning (ML) is another important AI technology for knowledge management. It allows the system to comb through vast amounts of data to discover patterns that would not be visible to humans and could identify correlations that suggests a cause.
AI tools will enable workers to extract relevant, actionable, and role-appropriate information out of the deluge of data generated by a plant, including both automated data and the tacit job-related knowledge hiding in employee logs and observations. One promising emerging tools is smart search, which allows people to query the system using natural language and returns results relevant to their role and the context of the question. Instead of sifting through mountains of irrelevant results from a keyword search, people can quickly find the exact information they need.
Another tool, solution suggestion goes a step further. These AI systems can pull information from several sources and analyse data using ML to uncover process similarities and patterns such as if the ‘product turned dark’ or the ‘fluid showed black spots’. Such pattern intricacies can be identified and provided to the decision-maker for determining how it will be handled. Tools like this are moving the chemical industry forward from Industry 4.0 (basic automation and digitisation) to Industry 5.0, amplifying the performance of people through technology.
A smart, centralised knowledge management system will be an essential component of knowledge transfer in the very near term. As experienced long-time manufacturing workers continue to retire at record rates, chemical manufacturers are experiencing a brain drain as institutional knowledge walks out the door. Today’s younger workers switch jobs, employers and even industries much more frequently, making it difficult for them to accumulate the job-specific experience they need to excel.
At the same time, manufacturers are running leaner, with fewer people managing more processes, systems and data. In generations past, tacit knowledge was transferred naturally and gradually, as new employees worked with more experienced colleagues, and younger workers had plenty of time to get up to speed with their roles.
To stay competitive today, manufacturers will need to expedite the knowledge transfer process. They will also need to ensure that knowledge from experienced workers is preserved and remains accessible after they are gone. An AI assist can help manufacturers solve the tacit knowledge problem and enable all employees to maximise their effectiveness, whether they have been on the job for five months, five years or twenty-five years.